Keynotes
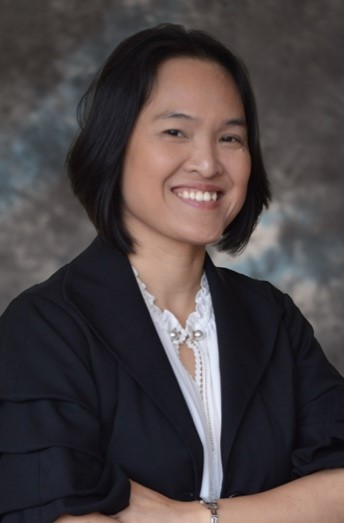
My T. Thai
Title: Interpretability and Privacy Preservation in Large Language Models (LLMs)
Bio:
My T. Thai is a University of Florida (UF) Research Foundation Professor, Associate Director of UF Nelms Institute for the Connected World, and a Fellow of IEEE and AAIA. Dr. Thai is a leading authority who has done transformative research in Trustworthy AI and Optimization, especially for complex systems with applications to healthcare, social media, critical networking infrastructure, and cybersecurity. The results of her work have led to 7 books and 350+ publications in highly ranked international journals and conferences, including several best paper awards from the IEEE, ACM, and AAAI.
In responding to a world-wide call of responsible and safety AI, Dr. Thai is a pioneer in designing deep explanations for black-box ML models, while defending against explanation-guided attacks, evident by her Distinguished Papers Award at the Association for the Advancement of Artificial Intelligence (AAAI) conference on AI, 2023. At the same year, she was also awarded an ACM Web Science Trust Test-of-Time award, for her landmark work on combating misinformation in social media. In 2022, she received an IEEE Big Data Security Women of Achievement Award. In 2009, she was awarded the Young Investigator (YIP) from the Defense Threat Reduction Agency (DTRA) and in 2010, she won the NSF CAREER Award. She is presently the Editor-in-Chief of Springer Journal of Combinatorial Optimization, IET Blockchain Journal, and book series editor of Springer Optimization and Its Applications.
Abstract:
Large Language Models (LLMs) have transformed the AI landscape, captivating researchers and practitioners with their remarkable ability to generate human-like text and perform complex tasks. However, this transformative power comes with a set of critical challenges, particularly in the realms of interpretability and privacy preservation. In this talk, we embark on an exploration of these pressing issues, shedding light on how LLMs operate, their limitations, and the strategies we can employ to mitigate risks. We begin by examining the interpretability in LLMs, which often function as enigmatic “black boxes.” Their complex neural architectures make it challenging to understand how they arrive at specific outputs. This lack of transparency raises questions of trust and accountability. When deploying LLMs in real-world applications—whether for chatbots, content generation, or decision-making—it becomes crucial to demystify their decision paths. We will use explainable AI (XAI) to offer faithful explanations, from the black-box to white-box models, and from feature-based to neuron circuits-based explanations. We next turn our attention to one of the utmost concerns and challenges: data privacy. LLMs process vast amounts of data, raising risks of data leakage, model inversion, the right to be forgotten, and inadvertent exposure of sensitive information. Furthermore, the integration of LLMs into diverse applications also significantly brings these challenges to the next level. This talk explores strategies to protect privacy, including differential privacy and federated learning.